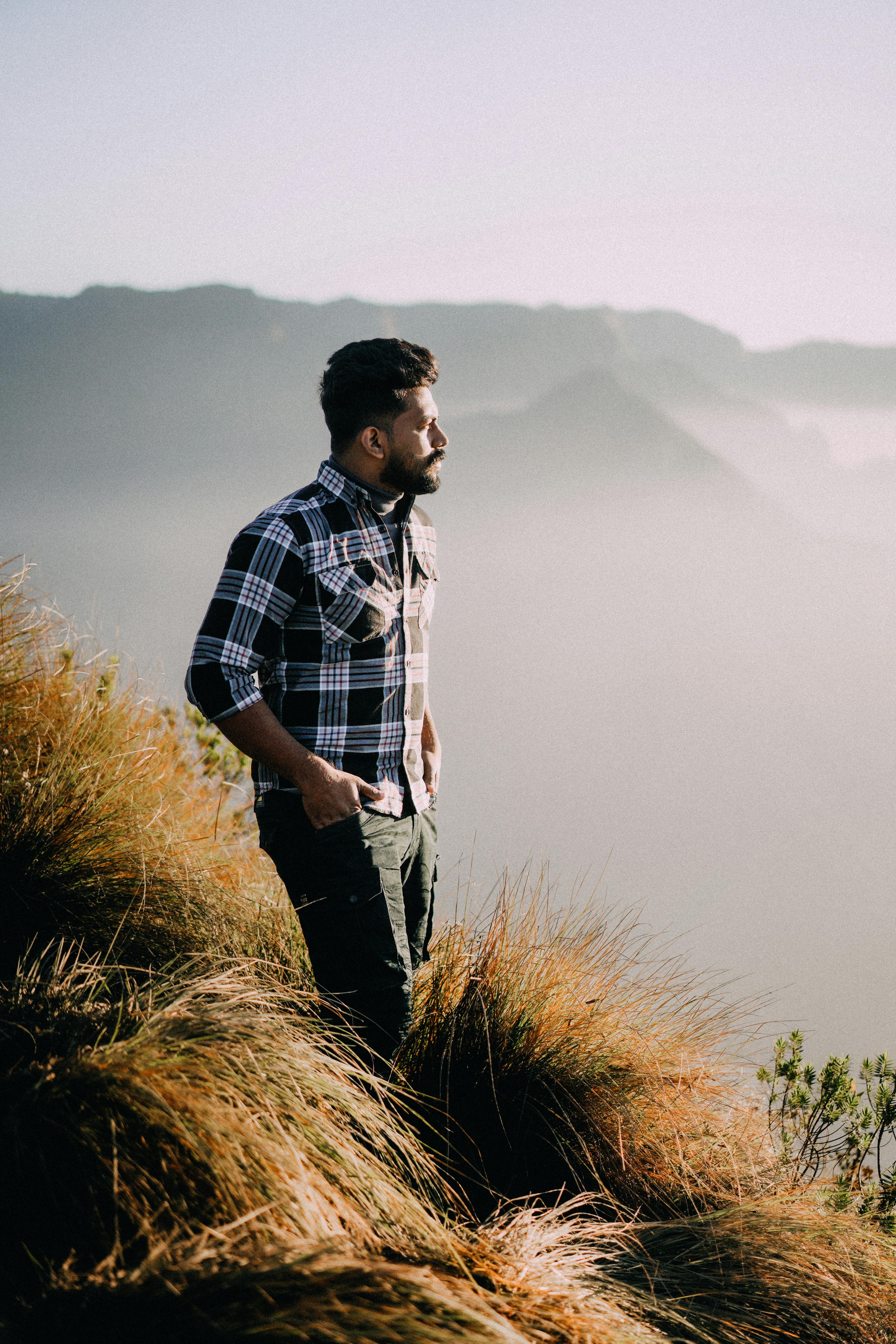
Practical Guide to How to Find Confidence Interval
Understanding Confidence Intervals in Statistical Inference
Confidence intervals are a fundamental concept in **statistical inference**, providing a range of values within which we expect the true population parameter to lie. The confidence interval is constructed based on a sample statistic, such as the **sample mean**, and takes into account variability within the data. For instance, if we have a sample mean of 50 and a margin of error of 5, our confidence interval would indicate that we are 95% confident that the true population mean lies between 45 and 55. The reliability of these estimates is heavily reliant on the chosen **confidence level**—typically set at 90%, 95%, or 99%—which reflects the degree of certainty within the interval estimate.
Components of a Confidence Interval
The establishment of a confidence interval involves several key components: the **point estimate**, which is usually the sample mean, plus or minus the margin of error. The margin of error itself can be computed using the **standard deviation** and a critical value that corresponds to the selected confidence level—often either from the **t-distribution** (for smaller sample sizes) or the **z-distribution** (for larger sample sizes). For example, the formula for calculating a confidence interval using the z-score is expressed as follows:
Confidence Interval = Sample Mean ± (Critical Value × Standard Error).
In practical terms, this means that for larger data sets where the **normal distribution** applies, we can derive more precise confidence limits.
Critical Values in Confidence Interval Calculation
**Critical values** are essential for determining the width of the confidence interval. For instance, in a z-distribution, the critical value for a 95% confidence level is 1.96, implying that if we take repeated samples, 95% of the intervals will capture the true population mean. For small samples where the population standard deviation is unknown, the critical value would be determined using the t-distribution, which accounts for increased variability as sample sizes decrease. Correct application is vital to ensuring that we maintain proper **statistical reliability** and reduce potential **measurement error** in our estimates.
Calculating Confidence Intervals: Step-by-Step
Finding the confidence interval is quite straightforward when following a systematic process. First, begin with your **data collection** to ensure adequate sample size is achieved. In statistical analysis, a sample size that is too small may lead to substantial errors in your confidence intervals. Next, you will calculate your sample mean and standard deviation. Once these statistics are obtained, it is time to determine the desired **confidence level** and its corresponding critical value. Let’s examine these steps closely.
Step 1: Gather Your Data
The initial step in creating your confidence interval is the process of gathering and analyzing your data. Employ **random sampling** techniques to enhance the representativeness of your sample. For instance, using **systematic sampling** can provide an efficient means of data collection, especially in large data sets. Take a central tendent statistic, such as the mean, to better understand where your observations lie relative to the overall population.
Step 2: Calculate the Margin of Error
After achieving your sample statistics, computation of the **margin of error** is next. This in part relies on the **standard error** of the mean calculated as Standard Deviation divided by the square root of the sample size. Hence, knowing the variability in your data is crucial. With the margin of error quantified, you can construct your confidence interval accurately. For example, if your calculated margin of error is 3, a sample mean of 20 would yield a confidence interval of (17, 23).
Common Estimation Techniques for Confidence Intervals
Several robust methodologies exist to solidify confidence interval calculations. Each technique varies based on the distributional assumptions of your data and the level of precision needed in your estimates. The two predominant methods—the **bootstrapping** technique and parametric approaches—are frequently used in practice to enhance inferential statistics.
Bootstrapping Confidence Intervals
**Bootstrapping** is a resampling technique that allows researchers to generate a sampling distribution by repeatedly drawing random samples with replacement from a dataset. This method is remarkably beneficial especially when the original data set is small. Bootstrapping produces multiple estimates of a statistic, which can then form a confidence interval by taking percentiles from the resulting distribution. For example, if the 2nd and 98th percentiles correspond to your estimates, those values will form the edges of your confidence interval, thus displaying a robust estimation method free from normality assumptions.
Parametric Approaches to Confidence Interval Calculation
Parametric approaches assume that the data fits a specific distribution, most commonly the **normal distribution**. Based on this assumption, confidence intervals can be derived using standard formulas which involve means and standard deviations. This approach, although powerful, should be categorized by careful scrutiny of the distribution shape through the use of tests such as the **Shapiro-Wilk test** to validate the normality. It embodies both ease and statistical description value when distributions hold strong to parametric assumptions.
FAQs on Confidence Intervals
1. What are confidence intervals used for?
**Confidence intervals** are primarily used in research to infer population parameters based on sample data. They provide a range of values within which the true population parameter, such as the population mean or proportion, is expected to lie with a specified confidence level. This gives researchers a quantitative method of expressing uncertainty regarding their estimates, thereby making them essential in both **quantitative analysis** and **statistical inference**.
2. How do you interpret a confidence interval?
Interpreting a confidence interval involves understanding its boundaries. For instance, if you have a 95% confidence interval of (10, 20) for a population mean, this means that you are 95% confident that the true population mean lies between 10 and 20. It suggests a **margin of error** and provides insight into the **variability** of the sample data concerning the parameter of interest.
3. What affects the width of a confidence interval?
The width of a confidence interval is influenced primarily by the **sample size** and the **confidence level** chosen. Greater sample sizes tend to lower the variability and lead to narrower intervals, offering more precise estimates. Conversely, selecting a higher confidence level (e.g., from 90% to 95% or 99%) will lead to wider confidence intervals, demonstrating an inherent trade-off between the precision of estimates and the desired reliability of statistical conclusions.
4. Can confidence intervals be negative?
Confidence intervals can indeed extend into negative values, especially when dealing with measures that can assume both positive and negative outcomes, such as differences between two means. However, the context will determine the relevance, as negative intervals can imply a lack of effect or correlation in many **hypothesis testing** scenarios, especially where relationships are tested. Interpretation must therefore assess the realities of the domain studied.
5. What is the significance of the central limit theorem in constructing confidence intervals?
The **central limit theorem** underpins the confidence interval's vital importance; it states that as the sample size increases, the sampling distribution of the sample mean will approach a normal distribution, no matter the population's original distribution. This theorem allows researchers to apply normal distribution principles when forming confidence intervals, even with non-normally distributed data, significantly bolstering the **accuracy measurement** across diverse practical applications.
Key Takeaways
- Confidence intervals provide important statistical estimates and are used to infer population parameters from sample statistics.
- Components such as sample mean, margin of error, and critical values are crucial for constructing confidence intervals.
- Methodologies like bootstrapping and parametric approaches offer reliable means of computing confidence intervals.
- Interpreting confidence intervals involves understanding their range and what it suggests about the population parameter.
If you're looking for deeper insights or further guidance on confidence intervals and statistical methodology, please reach out or explore additional resources available through our site.
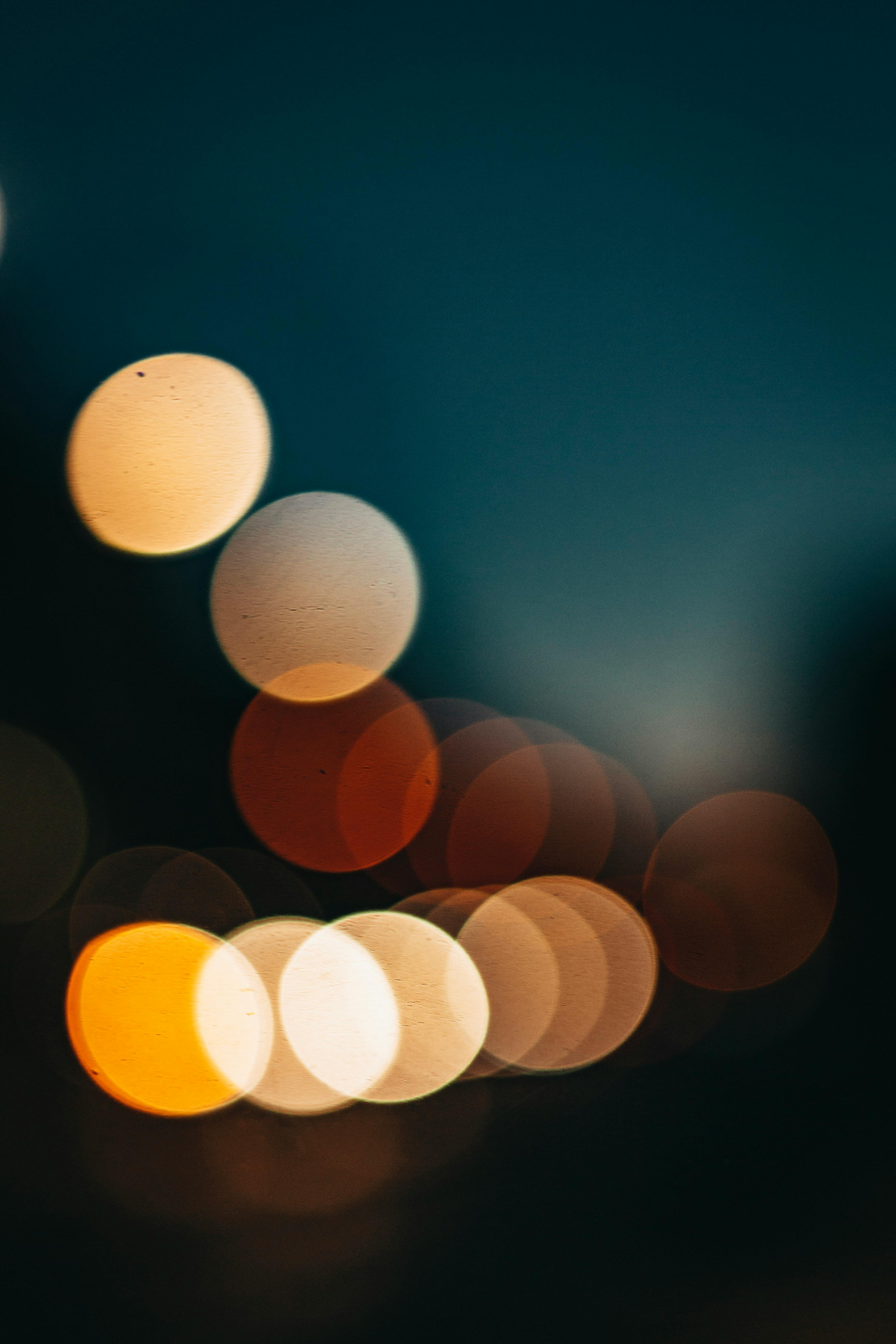
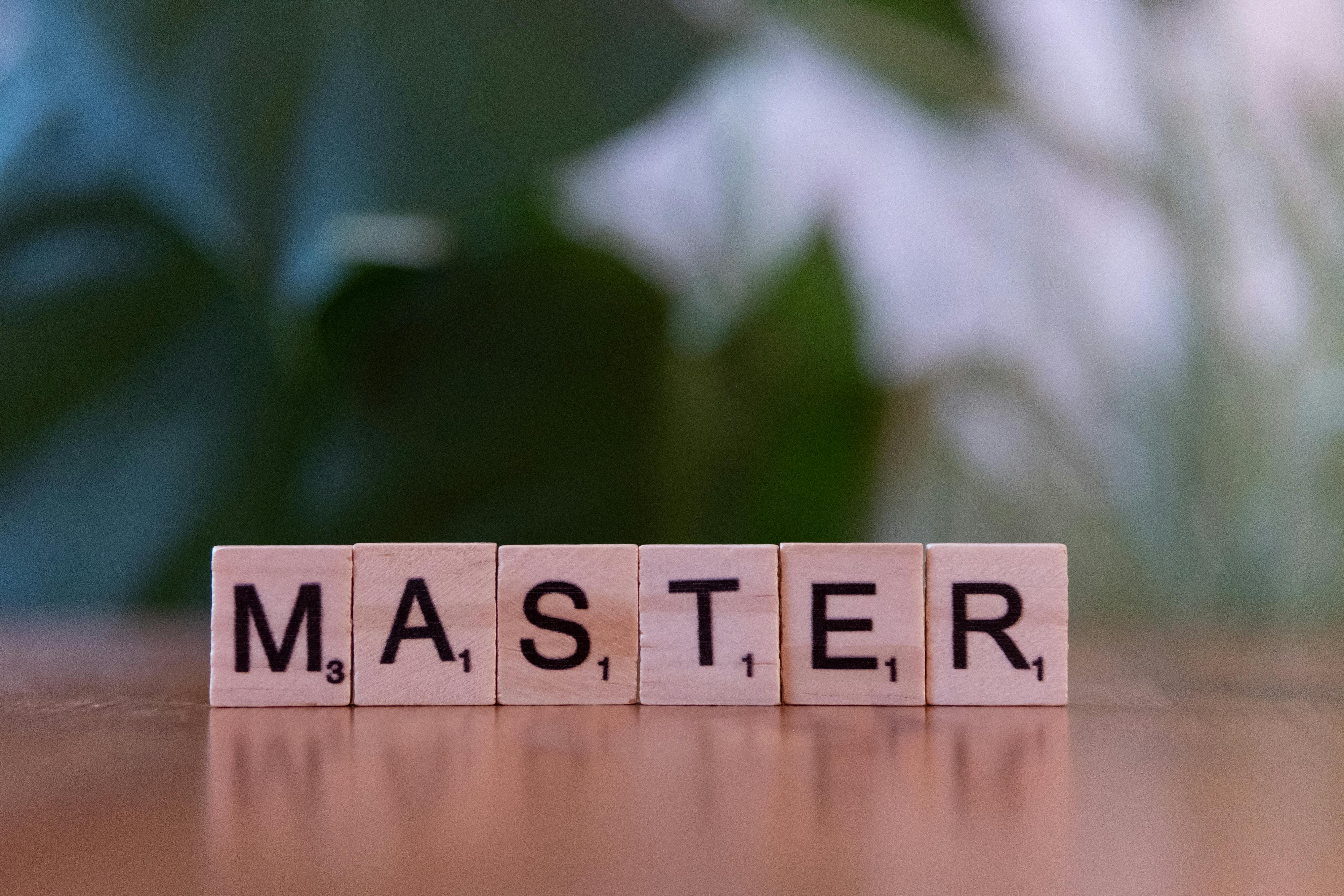