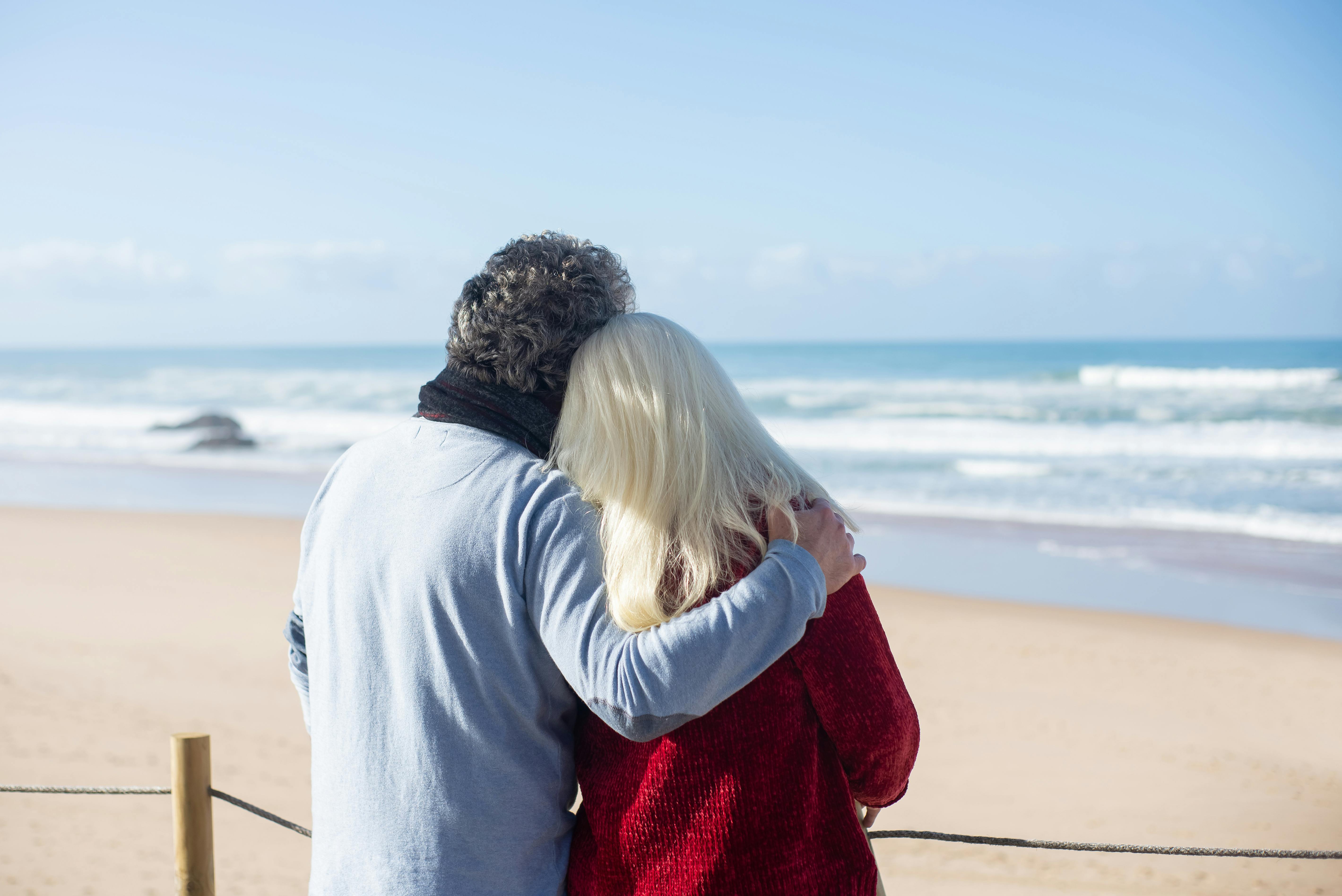
How to Effectively Find the IQR of Your Data Set in 2025
The interquartile range (IQR) is a fundamental concept in statistics used to measure the spread of data and identify outliers. Understanding how to find the IQR is essential for effective data analysis. In this guide, we will explain the process of calculating IQR using practical examples and advanced statistical methods. Whether you are just beginning to dive into data analysis or are already well-versed in statistics, this article will enhance your understanding of the IQR and its relevance in various applications.
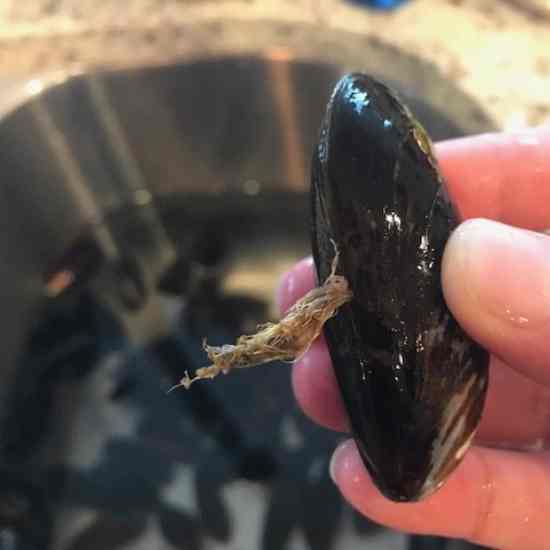
Understanding the IQR in Statistics
The interquartile range (IQR) represents the middle fifty percent of a data set. It is calculated as the difference between the first quartile (Q1) and the third quartile (Q3). The IQR provides a measure of statistical dispersion and helps to understand the **data spread**. Unlike the range, which considers only extreme values, the IQR focuses on the core distribution of the data, making it a robust measure of dispersion. This is especially useful when dealing with outliers since it is not affected by extreme values, providing a more accurate representation of the data variability.
Defining Interquartile Range
The IQR is defined as: IQR = Q3 - Q1, where Q1 is the first quartile (25th percentile) and Q3 is the third quartile (75th percentile). To find these quartiles, you can use different quartile calculation methods depending on the tool or software at your disposal. For instance, many statistical software packages and programming languages provide built-in functions to easily compute quartiles. It's crucial to understand that an accurate understanding of **quartiles** is fundamental to calculating the IQR correctly!
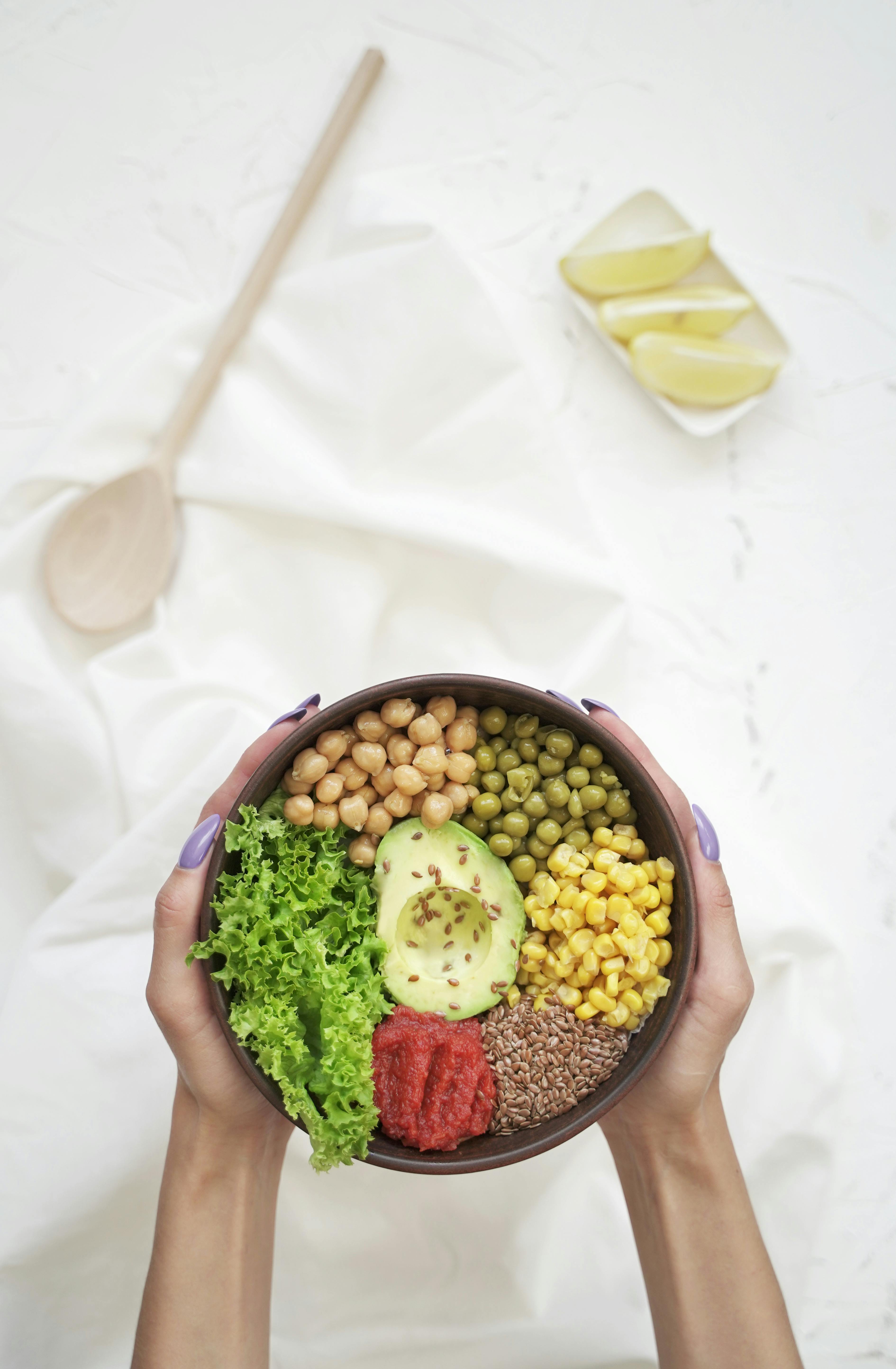
The Significance of IQR
The **significance of IQR** lies in its ability to provide insights into the underlying distribution of your data set. By measuring the central tendency and variability, the IQR acts as a robust statistic to help identify outliers. For instance, if you determine that a data point falls beyond Q1 - 1.5 * IQR or Q3 + 1.5 * IQR, it is typically classified as an outlier. This knowledge is critical for effective data analysis as it allows researchers to make data-driven decisions, enhancing interpretative accuracy in research studies.
Calculating IQR Step by Step
Understanding how to find the IQR can be simplified into a few clear steps. Whether you opt for manual calculations or use software tools, adhering to these processes ensures accuracy and consistency.
Step 1: Organizing Data
Begin by organizing your data in ascending order. This foundational step is crucial for effective **data analysis**. Without proper organization, determining the quartiles becomes challenging. For example, if your data set consists of the values: 3, 5, 2, 8, 7, we first sort it to get: 2, 3, 5, 7, 8. This will allow for easy identification of Q1 and Q3.
Step 2: Finding Quartiles
Next, calculate the first quartile (Q1) and the third quartile (Q3). The median divides your data set into two halves. Q1 is then found as the median of the lower half of the dataset, and Q3 as the median of the upper half. Using our sorted data of 2, 3, 5, 7, and 8, we find Q1 = 3 and Q3 = 7. Calculating \textbf{IQR} is then straightforward with this information!
Step 3: Calculating the IQR
Finally, subtract Q1 from Q3 to find the IQR: IQR = Q3 - Q1. In our example, IQR = 7 - 3 = 4. This IQR value provides insights into the **data distribution’s spread**, informing decisions about variability and outliers.
Visualizing IQR
Utilizing **box plots and IQR** representation is an effective statistical visualization technique. A box plot visually summarizes the key quartiles alongside outliers, giving a clear picture of data distribution.
Creating Box-and-Whisker Plots
To construct a box plot, draw a box from Q1 to Q3 and extend "whiskers" to the smallest and largest values within Q1 - 1.5 * IQR and Q3 + 1.5 * IQR. Any data points outside of this range represent potential outliers and stand separate in your plot. This visual approach not only enhances interpretation but is instrumental in showcasing **data outliers and IQR** efficiently.
Advantages of Using IQR
The advantages of IQR in statistical analysis include its robustness against outliers, clarity in data representation, and ease of computation. Using IQR is beneficial when conducting comparative studies, thereby enhancing your ability to draw valid conclusions from data sets. It promotes informed decision-making, particularly in research where precise data interpretation can significantly influence outcomes.
IQR in Research and Its Applications
Understanding how to apply the IQR can enhance data interpretation in various fields such as research, business, and social sciences. Recognizing the role of IQR in these domains equips statisticians with knowledge beneficial for engaging in **data-driven decisions**.
Interquartile Range in Research
In research, employing the IQR allows analysts to better understand data distributions and variability associated with study results. This is particularly useful in quantifying differences between groups or testing various statistical hypotheses. For instance, in clinical studies analyzing treatment effects, **measuring dispersion** using IQR is effective for framing the significance of outcomes.
Using IQR in Data Science
Within the realm of data science, the **importance of interquartile range** extends beyond simple measurements of spread. IQR provides a means to clean data efficiently by identifying and managing outliers. Thus, mastering IQR techniques is instrumental for any data science professional seeking to apply robust statistical methods within their analyses.
Key Takeaways
- The IQR is crucial for understanding data spread and isolating outliers effectively.
- Calculate IQR by finding Q1 and Q3 and applying the formula IQR = Q3 - Q1.
- Visualizing IQR using box plots aids in interpreting complex data distributions.
- The IQR is widely applicable across various research fields, enhancing data-driven decision-making.
FAQ
1. What is the IQR formula and how is it calculated?
The IQR formula is defined as IQR = Q3 - Q1, where Q1 is the first quartile and Q3 is the third quartile. To find these quartiles, order your data, find the median of the lower half for Q1, and the upper half for Q3, and then subtract the two values.
2. How does IQR compare to the range in statistics?
The range measures the difference between the highest and lowest values in a dataset, whereas the IQR focuses on the spread of the central half of the data. Thus, IQR provides a more robust indication of variability in the presence of outliers.
3. Can IQR help in identifying outliers?
Yes! Values that fall below Q1 - 1.5 * IQR or above Q3 + 1.5 * IQR are typically considered outliers. This property makes IQR a powerful tool for enhancing statistical analyses and clarifying data distributions.
4. What are the advantages of using IQR in data analysis?
The advantages of using IQR include its robustness against extreme values, effectiveness in visualizing data distribution through box plots, and reliability for identifying and managing outliers. This makes it invaluable in statistical and practical scenarios.
5. How can IQR be used in the field of research?
IQR is extensively used in research for analyzing the variance of data sets, facilitating the assessment of treatment effects and overall trends in various studies. In this manner, it allows for deeper statistical insight and more informed conclusions.