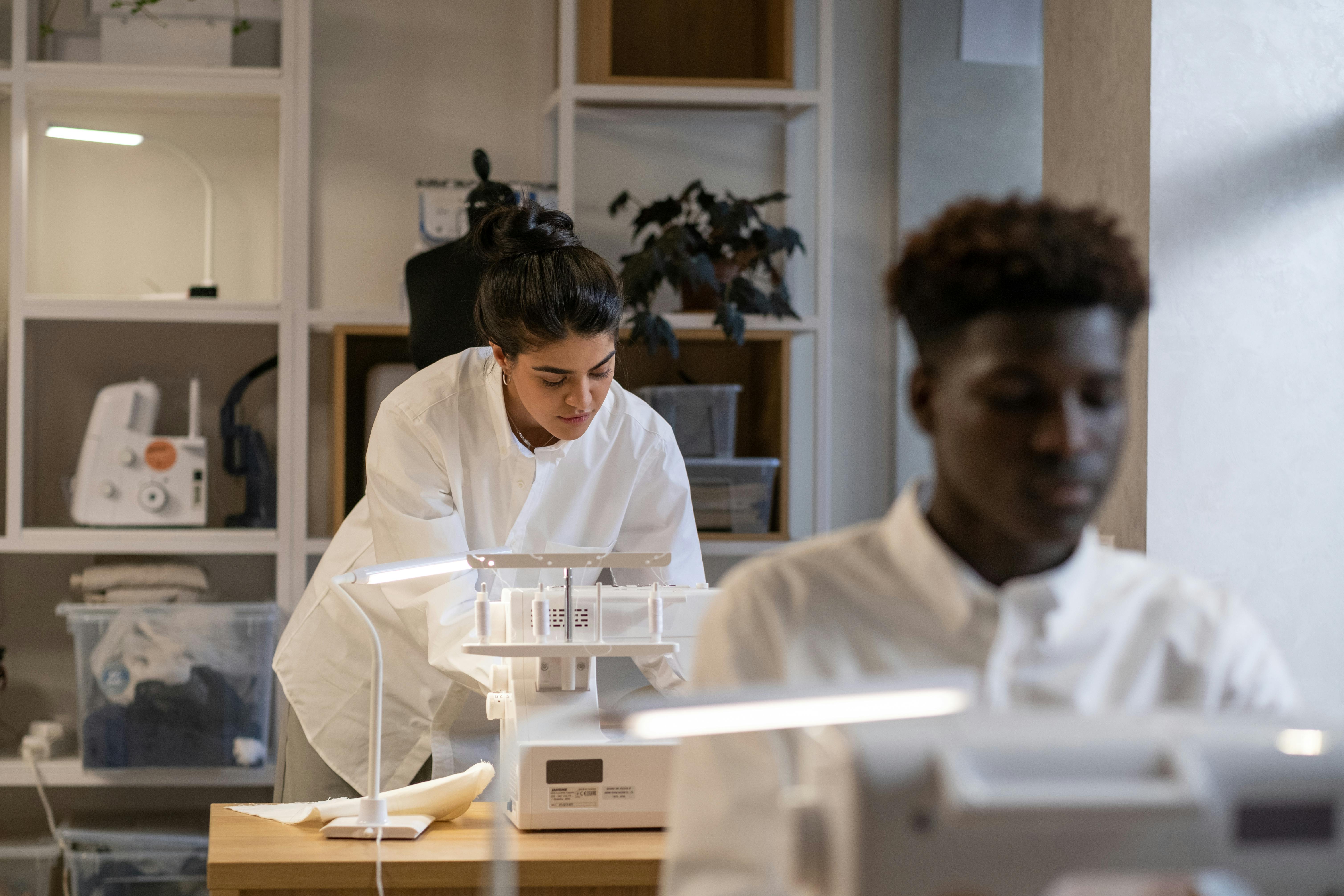
Effective Ways to Find Degrees of Freedom in Statistical Analysis
Understanding Degrees of Freedom in Statistical Analysis
In statistical analysis, **degrees of freedom** (df) are crucial for interpreting results, critical in determining the number of values in a statistical calculation that are free to vary. Generally, df is calculated by deducting the number of constraints from the total number of values considered, making it pivotal in methods like ***variance analysis***, **hypothesis testing**, and various statistical models. For instance, when applying the concepts of ANOVA (Analysis of Variance) or t-tests, degrees of freedom directly affect the critical value used to accept or reject the null hypothesis. With an understanding of df, researchers can ensure more accurate statistical significance results, presenting a clear picture of data variability and underlying patterns.
What Are Degrees of Freedom?
Degrees of freedom represent the number of independent ways in which a dynamic system can change. In the context of statistics, it denotes the number of independent values or quantities which can vary in the analysis without violating any given constraints. Specifically, in **variance analysis**, the degrees of freedom can affect the computations of sample variance, which is crucial when generalizing sample findings to broader population parameters. A well-established formula for calculating degrees of freedom in different contexts includes: df = n - k, where n represents the sample size and k the number of parameters estimated. Accurately determining degrees of freedom ensures justified application in **hypothesis testing** and aids in the applied research field.
Why Degrees of Freedom Matter in Statistical Tests
Utilizing degrees of freedom effectively is essential when evaluating statistical tests like ***t-tests***, **chi-squared tests**, and **ANOVA**. For instance, in applying a t-test, which involves comparing means between two groups, the degrees of freedom impact the critical value derived from the t-distribution table. Decreasing degrees of freedom can lead to wider confidence intervals, impacting **statistical significance**. Likewise, in **chi-squared tests**, df is vital for determining if observed frequencies significantly differ from expected frequencies in categorical data. Thus, mastery of how to calculate and apply degrees of freedom in **statistical methods** can enhance the accuracy and reliability of data interpretations and influence the robustness of research designs.
Methods for Determining Degrees of Freedom
Determining degrees of freedom can be approached in several ways depending on the statistical method being applied. Utilizing systematic and robust processes assists in ensuring **statistical reliability** and confidence in results. Here are some effective ways to compute degrees of freedom tailored for specific statistical tests.
Degrees of Freedom in t-Tests
For ***t-tests***, the degrees of freedom can be derived as follows: for independent samples, df = (n1 - 1) + (n2 - 1), where n1 and n2 are the sizes of the two samples. This methodology emphasizes how many independent observations were available to estimate the population parameters accurately. A working knowledge of this allows researchers to effectively apply **hypothesis formulation** and grasp the inherent variability of different samples. When conducting paired samples t-tests, the calculation is simpler and is given by df = n - 1, where n refers to the number of pairs analyzed. Mastering the application of these methodologies advances a researcher's statistical analysis capabilities.
Degrees of Freedom in ANOVA
Within the framework of **ANOVA**, calculating degrees of freedom involves two distinct components: the between-group and within-group degrees of freedom. The formula for between-group df is k - 1 (k = number of groups), and within-group df is N - k (N = total number of observations). Cumulatively, this computation not only advances the understanding of group variability but also facilitates effective studies in factors like **experimental design** and statistical power. When researchers grasp these calculations, they can significantly improve their capabilities in performing **variance analysis** and determining the significance of predictor variables in their models.
Using Chi-Squared Tests for Independence
In employing chi-squared tests, particularly for independence, degrees of freedom are calculated by df = (r - 1)(c - 1), where r represents the number of rows and c represents the number of columns in the contingency table. Understanding and applying this formula is not merely an academic exercise; it enhances the analysis of relationships among variables, supports the assessment of **independence tests**, and bolsters the overall accuracy of inference. By mastering chi-squared tests and degrees of freedom concepts, researchers gain robust tools to identify patterns within their data sets readily.
Practical Application of Degrees of Freedom in Statistical Analysis
Having a firm understanding of how to determine degrees of freedom has practical implications across numerous domains, impacting the effectiveness and accuracy of statistical analysis in a range of applications. Such analytical capabilities are paramount in executing various quantitative research methodologies.
Integrating Degrees of Freedom Into Hypothesis Testing
The incorporation of degrees of freedom into **hypothesis testing** forms the backbone of statistical inference. By acknowledging the number of allowable values for a statistical application, researchers enhance their degree of confidence in negative or positive results, thus improving **statistical significance** judgments. Using insights from degrees of freedom, analysts can deliberately shape their research designs to test hypotheses more effectively, setting the groundwork for impactful empirical research.
Using Degrees of Freedom for Model Fitting
Efficient **model fitting** requires careful consideration of degrees of freedom, particularly when analyzing regression datasets. Each predictor variable included in the model reduces the degrees of freedom available to assess the model fit, emphasizing the importance of balancing simplicity with accuracy. Failure to account for this balance can culminate in poor model performance and diminished **predictive accuracy**. Therefore, ensuring an adept selection of variables while keeping track of their effect on degree calculations can lead to more accurate interpretations and findings.
Degrees of Freedom in Experimental Design and Reliability Analysis
Degrees of freedom play a pragmatic role in **experimental design**, particularly in factorial designs that require careful control of variables to yield reliable results. Understanding how different factors interact, alongside employing degrees of freedom for analysis, facilitates robust judgments about **data variability** and promotes enhanced **reliability analysis** to draw valid conclusions. This systematic approach greatly improves data's integrity and fosters robust experimental outcomes.
Key Takeaways
- Degrees of freedom are essential for proper interpretation of statistical tests and models.
- Methods for calculating degrees of freedom vary with the statistical approach used.
- Incorporating the correct degrees of freedom can significantly impact the outcome of hypothesis testing.
- Proper use of degrees of freedom yields profound enhancements in data interpretation and research reliability.
- Mastering degrees of freedom boosts capabilities in empirical and inferential statistical analysis.
FAQ
1. What is the formula for calculating degrees of freedom?
The formula for calculating degrees of freedom generally depends on the statistical test being employed. For t-tests, degrees of freedom are calculated as df = n - 1 for single-sample t-tests, while for independent two-sample t-tests, it is df = (n1 - 1) + (n2 - 1). Understanding these formulas is vital for accurate hypothesis testing and statistical analysis across various study designs.
2. How do degrees of freedom affect hypothesis testing?
Degrees of freedom provide vital context for determining the critical values needed for hypothesis testing. They help define the distribution curve used in analysis. Higher degrees of freedom often lead to narrower confidence intervals, influencing the determination of techniques such as t-tests or ANOVA. This stems from their ability to reflect how much data contributes to the estimation of parameters, leading to more nuanced interpretations of results.
3. Why is understanding degrees of freedom important in ANOVA?
In **ANOVA**, understanding degrees of freedom is instrumental in assessing group variance. It helps researchers identify how many levels exist between different group means and aids in determining the significance of those differences. This comprehension ensures accurate interpretations that reflect true variance in experimental data, thereby facilitating effective decision-making based on statistical outcomes.
4. Can degrees of freedom be negative?
No, degrees of freedom cannot be negative as they represent a count of independent values. A negative value occurs due to a misalignment of calculations or parameters in statistical analysis. Accurate calculations rely on adjusting the sample size or accounting for constraints to ensure proper degrees of freedom calculation, vital for reliable scientific conclusions.
5. How can software assist in calculating degrees of freedom?
Statistical software automates the process of calculating degrees of freedom across various tests. By entering data sets and selecting appropriate statistical methods, researchers can swiftly obtain degrees of freedom alongside other vital outputs such as p-values and effect sizes. This speeds up the analysis process and mitigates calculation errors that may occur with manual computations.
6. What role do degrees of freedom play in statistical significance testing?
Degrees of freedom directly influence the critical values and p-values obtained from statistical tests. By accurately determining degrees of freedom, researchers can ensure their statistical significances reflect true relationships within the data. This enhances the reliability of the conclusions drawn, impacting practical significance and decision-making based on the statistical findings throughout empirical research.
7. How can researchers improve their understanding of degrees of freedom?
Researchers can enhance their understanding of degrees of freedom through practical exploration, attending workshops, and utilizing statistical software that provides detailed insights. Additionally, engaging with textbooks focused on **statistical theory** and practical applications assists in rounding out their knowledge on calculating and interpreting degrees of freedom across diverse statistical tests.